Artificial Intelligence Roadmap
< Back to AI Roadmap Landing Page
3.1.2 Societal Drivers for Integrated Intelligence
3.1.3 The Science of Integrated Intelligence
3.1.4 Contextualized AI
3.1.5 Open Knowledge Repositories
3.1.6 Understanding Human Intelligence
3. Overview of Core Technical Areas of AI Research Roadmap
As discussed in section 1.2 the CCC, with the support of AAAI, held three community workshops in order to catalyze discussion and generate this research Roadmap. The three workshops were:
The research priorities from the three areas are summarized in Figure 3 below and expanded upon in the following three sections of the report.
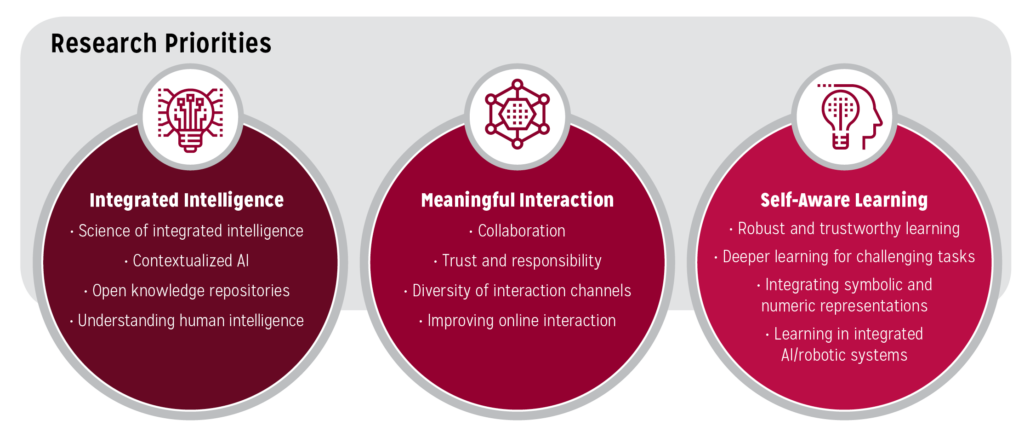
Figure 3. Research Priorities.
3.1 A Research Roadmap for Integrated Intelligence
3.1.1 Introduction and Overview
The development of integrated intelligent systems will require major research efforts, which we group into three major areas:
- Integration: Science of Integrated Intelligence will explore how to create intelligent systems that have much broader capabilities than today’s systems. Approaches include finding small sets of primitives out of which a broad range of capabilities can be constructed (like many of today’s cognitive architectures) and composing independently developed AI capabilities (like many of today’s deployed intelligent systems). Developing theoretical frameworks that provide analyses of performance and reliability will help us scale up and deploy more capable intelligent systems.
- Contextualization: Contextualized AI refers to the ability to adapt general AI capabilities to particular individuals, organizations, or functional roles (e.g., assistant vs coach). This requires both the ability to easily incorporate additional idiosyncratic knowledge without undermining off-the-shelf capabilities, and the ability for the systems to maintain and extend themselves by continuous adaptation to their context and circumstances. This will enable the creation of lifelong personal assistants whose data belongs to their owner, not a third party, and are able to build relationships over time with the people that they work with.
- Knowledge: Open knowledge repositories are needed to provide access to the vast amount of knowledge that is required to operate in the rich world we live in. The availability of open knowledge repositories will facilitate the development of a new generation of AI systems that can understand how the world works and behave accordingly.
This section begins with motivating vignettes for each of the societal drivers, highlighting the research required in each of these three areas, it then poses both stretch goals for the 2040 time frame and milestones to track progress along the way.
3.1.2 Societal Drivers for Integrated Intelligence
The vignettes below illustrate with concrete example the impacts across human society that would be made possible by AI systems that have integrated intelligence in the next twenty years.
Enhance Health and Quality of Life
Research Challenges. These visions will require a number of breakthroughs in AI in the three areas mentioned above:
- Integration: The complexity and criticality of the diverse capabilities involved in these scenarios will require well-engineered AI systems with assurances of their performance and predictability of their behaviors.
- Contextualization: AI systems will need to understand how each individual lives in a different environment with particular health needs, lifestyle choices, household situations, and social settings. AI systems will need to adapt their initial knowledge over years or decades in order to fit the idiosyncratic lives of the individuals they serve, the people they know, and the ever-changing world around them.
- Knowledge: Access to broad knowledge about routine activities and common pursuits, about expectations in social interactions, and generally how the world works will be crucial to developing such assistants. They also will require knowledge about human intelligence and capabilities, about the kinds of limitations that disease and aging pose on those capabilities, and what physical and emotional support and coping mechanisms provide effective assistance. Extensive, up-to-date, reliable sources of state-of-the-art medical research will make them more capable, along with advanced reasoning capabilities that can deploy this knowledge in support of their owners’ health and well being.
Accelerate Scientific Discovery
Research Challenges. AI systems for scientific problem solving will have a significant impact in the future by supporting individuals, groups, and government agencies in scientific problem solving, decision making, research, and innovation. These systems will function as problem-solving amplifiers. Specific AI challenges involve:
- Integration: AI systems for scientific discovery will need to seamlessly integrate human language processing, reasoning, planning, and decision making capabilities in order to read the literature and generate hypotheses about the data at hand. These capabilities need to be integrated with robotic sensors and actuators for designing and executing effective scientific experiments.
- Contextualization: Although AI systems can have general knowledge and strategies for scientific discovery, each research problem requires identifying and incorporating specific information about the situation and updating it based on ongoing discoveries. In addition, in order to work effectively with diverse teams of scientists, AI systems will need to understand the expertise that each scientist brings to bear to the problem, and communicate information to each accordingly.
- Knowledge: AI systems will need to automatically extract and integrate knowledge from the ever-expanding published literature. Supporting science (and science-policy) collaborations will also entail sophisticated knowledge of teamwork and human-computer interaction. Causal reasoning, a critical component of scientific thought, is still a nascent area of AI research.
Research Challenges. Creating systems that can be deployed across a wide range of education and training contexts to improve people’s lives will require substantial advances in a number of areas of AI, especially:
- Integration: In addition to traditional intelligence capabilities such as sensing, planning, and problem solving, AI training systems will need sophisticated skills such as collaboration, creativity, and critical thinking. These will be crucial in helping learners gain the knowledge and skills for a modern workforce that must generate non-routine creative solutions to challenging problems.
- Contextualization: Since each person has a different background, topical interests, and ways of learning, it is essential that AI training systems accurately assess a learner’s current knowledge state and design appropriate teaching strategies based on that state. AI training systems will need to optimize topics, support personalized individual and group projects, and provide social networks and resources that create unique and effective opportunities for individual students.
- Knowledge: AI training systems will need extensive knowledge of traditional topics, including math, science, language arts, and humanities, as well as advanced technical topics such as business processes and machinery. In addition, they will require knowledge about how to connect those technical topics with the real world and the ability to generate rich scenarios and examples to effectively scaffold lessons.
Research Challenges.
- Integration: A wide range of intelligent capabilities will need to be combined to attain this type of functionality. Deep language understanding and extensive reasoning will be needed to automatically process news stories and other information sources, including weeding out inaccuracies and deliberate misinformation.
- Contextualization: AI advisory systems will need to develop detailed knowledge of their organizations, creating an institutional memory to inform future decisions that are suited to the particular ways in which the organization works in practice.
- Knowledge: AI advisory systems for businesses will require massive knowledge that covers history, geography, politics, and economics, among many other areas. They will also need extensive knowledge about the structure and operations of organizations and their subsystems (e.g., supply chains). A key capability will be to make plausible inferences about the potential effects of current events on an organization. This will require accurate causal reasoning about consequences, including using historical precedents to help evaluate the plausibility of a predicted outcome and warn of potential pitfalls.
3.1.3 The Science of Integrated Intelligence
Most AI research focuses on single techniques or families of techniques that share a common knowledge representation and are applied to isolated problems. However, there is increasing awareness that more advanced intelligent systems will require multiple forms of knowledge, reasoning, and learning, and will involve combinations of reactivity, deliberation, and reflection. These capabilities will be needed to build interactive systems, both cyber and physically embodied, that operate in uncertain environments and communicate with people. While we have ways to develop these individual capabilities, we lack an understanding or science of how to build systems that integrate them. A key open question is what is the best overall organizational approach: homogenous, non-modular systems; fixed, static modular organizations; dynamically modular systems that can be reconfigured over time; or some other variation. Beyond the overall organizational approach, there are also challenges in translation or co-existence of alternative knowledge representations, such as symbolic, statistical, and neural/distributed. Finally, we do not yet understand how to best design the overall control structure for integrated systems: that is, how an AI system can manage both sequential and parallel processes, and the extent to which control occurs in a top-down, goal-driven manner versus a bottom-up, data-driven manner. To achieve intelligent behavior, components will need to exchange rich information and coordinate their behaviors in a harmonious way, despite their expected interdependencies with other components.
To address these questions, we need a Science of Integration, focused in particular on integrated intelligence. A Science of Integration would define the space of possible organizations, possibly leveraging a formal language for specifying how systems are organized, similar to early work in computer architecture. The value of such a science is that it could support abstract and formal analysis, comparison, classification, and synthesis of integration approaches. It could provide a framework for both formal and empirical analysis of alternative methods. Such a framework would offer great benefits to researchers as a foundation for the rich systems required to perform the tasks of future AI.
Across the space of possible system organizations, AI systems will need to store and retrieve knowledge: that is, memory is fundamental to their function. In integrated systems, a unified shared memory enables individual components to share information and interoperate, as when knowledge of a play helps one understand how to interpret the actions of individual characters on stage. In turn, both memory and reasoning depend on the knowledge that they manipulate.
Components of Intelligence
Intelligent behaviors can be very sophisticated and complex. What are the main components and capabilities that produce these behaviors? A significant portion of AI research over the years has been inspired by the study of human intelligence, producing AI systems that include components of intelligence such as reasoning, problem solving, planning, learning, acting, reacting, understanding and generating language, collaborating, etc. At the same time, since human intelligence is not yet well understood, many AI researchers have focused on engineering new approaches that solve intelligent tasks in ways that had little connection with human intelligence, effectively creating new components of intelligence. This has created an enormous heterogeneity in both the design and functionality of these components. It is very challenging to compare and contrast the capabilities of a given component unless it can be characterized and compared in terms of the intelligent behaviors it supports.
AI systems should, ideally, be created to have minimum complexity while satisfying their design requirements. Associated research challenges include how to specify requirements for AI systems, how to map those requirements into intelligent components, and how to select appropriate architectures that can accommodate those components. Designing the effective and parsimonious AI systems of the future would be greatly facilitated if such modular approaches to integrated intelligence were possible.
Much is known in neuroscience about the structure of the brain at the anatomical and functional levels. The human brain combines specialized, modular structures with broad distributed connectivity. Brain modules do not generally correspond to the components of intelligence adopted by AI and often interact at a finer temporal grain scale. Resolving discrepancies between AI and neuroscience perspectives on the nature and interaction of the components of intelligence could bootstrap a more productive relation between the two fields.
Stretch goals: By 2040, AI systems will integrate a variety of intelligent components and capabilities for adaptive low-level and high-level reasoning in complex environments. Milestones along this path include—
5 years: AI systems can be created by identifying what major intelligent capabilities are needed, and then selecting and configuring appropriate off-the-shelf components.
10 years: AI systems will combine a broad spectrum of components and learning mechanisms.
15 years: AI systems will handle new and unexpected situations gracefully by resorting to first principles, analogies, causal reasoning, and other creative processes.
Memory
The success of future AI systems will depend on developing new approaches to memory. For example, future intelligent personalized assistants will have to retain and organize memories that are lifelong and life-wide. This presents challenges for computer scientists, behavioral researchers, educators, and ethicists. Lifelong systems will require the capability to organize, synthesize, and retrieve large quantities of information in meaningful ways. Life-wide systems will need to retrieve across contexts and to support a spectrum of tasks. Because some system tasks and task environments will be unknown when the systems are first fielded, these AI system will need to adapt, reorganizing their memories and developing new retrieval schemes.
Although many learning approaches can be seen as building up memories from their training, the associated storage is often narrowly tuned for specific tasks, storing only generalizations, rather than the examples themselves. Capturing and retaining rich episodes that augment examples with their context will enable one-shot analogical learning and will increase flexibility. It will also increase explainability, by enabling systems to point to intelligible factors underlying their decisions. To provide this functionality, memory technologies for future AI systems will need to recognize and retrieve near-miss examples, to retrieve relevant information across different domains, to generalize in appropriate ways, and to re-organize the contents of their memory as new information is encountered. They will also need to integrate this memory with other processes—for example, using stored experiences to provide expectations for an intelligent agent to guide its understanding of a new phenomenon.
Human memory is perhaps the most thoroughly studied system in cognitive science, with its capabilities and limitations the subject of systematic experimental studies. Human memory relies on a hierarchical system of working memory, episodic memory, and semantic memory that have distinct, complementary characteristics in terms of time span and generalization. Working memory provides limited, efficient storage of local task context; episodic memory stores the rich, specific multimodal episodes that provide the basis of our identity; while semantic memory abstracts and generalizes knowledge for broad, long-term use in a variety of contexts. Because of capacity and access limitations (not unlike those of, say, robotic systems) human memory has evolved mechanisms to efficiently store a lifetime of information in constantly changing, uncertain environments and heuristically access it in real time under severe task constraints.
Memories captured and used by future AI systems will range from those that are specific to a particular individual at a particular time, to population-wide event and cultural memories, to metadata about all levels of a massive structure of varied, interconnecting topics. Humans now make extensive use of external knowledge sources, in multimodal formats that include text, video, and images. In order for AI systems to achieve coverage on a par with—or beyond—humans, and to be able to understand the knowledge of humans with whom they interact, future memory systems will need to exploit the enormous digital knowledge sources that now exist.
As AI systems build up new knowledge from interactions with people and other sources, they will need to assess the reasonableness of, and set limits to, the knowledge that they capture and queries they serve. Both mined information (e.g., on social media), and the memories they reconstruct may be inaccurate. AI systems will need to manage conflicting information, both at storage and reconstruction time. For example, AI research assistants will need to ingest and understand vast amounts of scientific literature, including experimental results that may sometimes conflict. More generally, they will need to reason about their own knowledge, in order to “know what they know, or don’t know,” to provide information with confidence when warranted and appropriate caution when not, and to guide the search for new knowledge.
Stretch goals: By 2040, AI memory systems will provide lifelong and life-wide repositories of experiences and other information, with fully context-aware and task-relevant retrieval. They will integrate the experience of systems and information captured from many sources, including the experiences of people captured by a wide range of heterogeneous, vast external multimodal sources. These memory systems will be able to flexibly reorganize themselves and reconstruct information as needed to appropriately exploit knowledge available across different systems. They will reason about the limitations of their own processes and knowledge, enabling people and AI systems to understand those limitations and uncertainties. The security, privacy, and sharing needs for individuals, institutions, and groups will be embedded in the structure of these systems. Milestones along this path include—
5 years: AI memory systems will be able to integrate and retrieve knowledge across tasks, knowledge types, and levels of abstraction, with active inference and tracking of uncertainties associated with observed and inferred information.
10 years: AI memory systems will flexibly assemble information from multiple sources, assess the quality of the knowledge from each source, and identify gaps.
15 years: AI memory systems will be easily reconfigured for new tasks and will integrate/assess multiple vast information sources, maintaining a clear distinction between bedrock memories, reconstructed plausible memories, and imaginings for the future.
Metareasoning and Reflection
Today’s high-performance AI systems require hundreds to thousands of human engineers to build, maintain, tune, and extend them. To achieve the goal of creating lifelong personal AIs, we need to learn how to make AI systems that do self-maintenance. This will require them to build and use models of themselves and data about their performance. Already, some cognitive architectures accumulate statistical metadata about their own knowledge for the purposes of estimating its utility and so better deploy it in new problems. More advanced metaknowledge will also include the ability to prioritize goals and estimate the timelines to achieve them and the likelihood of success. More accurate metaknowledge will enable AI systems to balance their workloads better, including trading off effectively between responding to their users and investing in learning to do their jobs better.
Stretch goals: By 2040, metaknowledge and self-knowledge models will be robust enough to scaffold AI systems that maintain themselves over years of operation, learning continually and adapting to new challenges with little to no intervention by human designers. Milestones along this path include—
5 years: The need for support staff in maintaining and extending AI systems will be significantly reduced due to the ability for the systems themselves to take on more of the support burden. At least 20 percent of the maintenance and extensions will occur via user interaction with the system, rather than through redesign and reimplementation by an AI engineer.
10 years: Support staff will be required only for periodic maintenance, with autonomous operation and updates otherwise, including balancing activities for self-improvement with effective collaboration. At least 80 percent of maintenance and extensions will occur via routine user interaction with the system.
15 years: No routine manual inspections and checkups of AI systems will be needed; instead, support staff will be used on demand, when requested (infrequently) by either the system or the people working with it.
3.1.4 Contextualized AI
Contextualization refers to the adaptation of general intelligent capabilities for a particular individual, organization, situation, or purpose. This includes contextual knowledge about the world, historical context of the circumstances of that particular entity, and its circumstances as it interacts with other entities and with the world. Contextualization is necessary in order to develop AI systems that assist people in their daily lives at work and home, for leisure and for learning. It is also necessary for the development of AI systems that are tailored to the culture and processes of specific organizations. Contextualization will allow AI systems to be individual, in that they use data and yet remain the property of their owner, and also be personalized, in that they prioritize the interests of the people interacting with them rather than third parties (e.g., their manufacturers). Contextualization will also allow AI systems to identify what sort of language is most familiar to their owner, which feedback improves a person’s well-being, and which approaches are most engaging. It will also support effective human-system collaboration, automated support of high-quality teamwork and rapid execution, and reasoning about social context and the social consequences of actions.
Customization of General Capabilities
AI systems with a broad range of intelligent capabilities will need to be personalized to an individual, tailored to an organization or group, redirected to particular purposes, and adapted to changes in the environment where they operate. To accomplish this, off-the-shelf, general AI components will need to be transformed in appropriate ways to operate in their particular contexts. Today, many AI systems can be customized, but only in very narrow ways. For example, current personal assistants (e.g., Alexa) can learn a few preferences based on data from user interactions. However, they cannot take instructions about how a user would like them to behave, or assist a user differently in different contexts (their role as a parent at home, or a manager at work, or a gym member), or understand when the same preference applies in different contexts—or not.
The need for AI systems to adapt to their context has many dimensions, best exemplified by personal assistants. To be effective, these will require: 1) lifelong adaptation, supporting a user for the long haul through different stages of life; 2) life-wide adaptation, supporting all aspects of life at work and at home, for leisure and for learning; and 3) continuity, determining which customizations are relevant and incorporating them into new AI systems that a user may adopt later on.
Stretch goals: By 2040, AI systems that start with general abilities and over time can adapt effectively to specific users, organizations, or purposes. These adaptations will continue over time as their environment changes, will be coordinated across tasks and activities, and will be transferable to new AI systems. Milestones along this path include—
5 years: AI systems will be able to extend their initially given knowledge and behaviors to fit into a particular environment.
10 years: AI systems will adapt their initial behaviors over long periods of time to reflect the changing situations and environments around them.
15 years: AI systems will be able to use any acquired preferences and customizations and adapt them for new tasks and goals.
Social Cognition
Social cognition seeks to understand interactions between individuals and how they understand each other. This has been a topic of study in multiple areas of cognitive science (e.g., psychology, linguistics, anthropology, neuroscience). To create AI systems that work effectively with people as collaborators, the systems must themselves be capable social beings, able to participate in social interactions. This will require significant advances in the understanding of social cognition from a computational perspective. The rest of this section outlines some relevant key areas.
To begin, AI systems will need to align with human values and norms to ensure that they behave ethically. They will need to take into account potential risks, benefits, harms, and costs. In order to do this, AI systems will have to incorporate complex ethical and commonsense reasoning capabilities that are needed to reliably and flexibly exhibit ethical behavior in a wide variety of interaction and decision making situations. They will potentially also be able to encourage humans toward ethical behaviors and even encourage respectful behaviors and decision making.
Finally, AI systems will need to build models of others as independent, intelligent beings. This will require having a model of their knowledge, their capabilities, their goals, and their awareness of the situation at hand. It will also require an awareness of how another human or AI system might, in turn, be modeling others.
Stretch goals: By 2040, AI systems will be capable of reasoning about social context, with behaviors appropriate to social events, awareness of people’s emotional states and reactions, and acknowledgment of shared and differing goals and reactions. Milestones along this path include—
5 years: AI systems will take social norms and contextual information into consideration when deciding how to pursue their goals.
10 years: AI systems will effectively reason about how to behave when faced with conflicting social norms and goals.
15 years: AI systems will handle situations where the motivations and goals of others interfere with accomplishing tasks, responding in appropriate ways: by changing their own tasks or negotiating with others.
3.1.5 Open Knowledge Repositories
AI researchers have long acknowledged the vast amount of knowledge about the world that humans acquire continuously from birth. This kind of knowledge, which is currently very difficult to incorporate into AI systems, includes understanding how people behave, commonsense knowledge about the physical world, and encyclopedic knowledge about objects, people, and other entities in the world.
Open knowledge repositories with massive amounts of world knowledge could fuel the next wave of knowledge-powered AI innovations, with transformative effects ranging from innovations in scientific research to the commercial sector. These knowledge resources could enable new research in machine learning systems that take human background knowledge into account, natural language systems that link words and sentences to meaningful descriptions, and robotics devices that operate with effective context and world knowledge. These open knowledge repositories could be extended to include domains of societal interest such as science, engineering, finance, and education. Through these systems, scientific research could exploit a vast trove of knowledge to augment data and support interdisciplinary research.
Industry is already benefiting from rich knowledge repositories. As technology companies push the envelope in markets such as search, question answering, entertainment, and advertising, they have tapped into the power afforded by massive amounts of knowledge about the world to make their systems ever more capable. This knowledge is typically captured as a graph containing entities of interest (e.g., products, organizations, notable people) interlinked in diverse dimensions and highly structured to enable abstraction and generalization. Currently, these knowledge bases focus on information particular to ecommerce and web search (e.g., information about products and geography), and do not include other kinds of knowledge about the world that are important for intelligent systems more broadly. Most are not openly available to academia, government, or smaller businesses.
Open knowledge repositories have the potential to impact science, education, and business if we mount an open effort to develop and expand shared resources. For example, already open knowledge repositories are ubiquitous in biomedical research. They represent entities of interest such as genes, proteins, organisms, diseases, and many other entities referenced and reasoned about in biomedical research. These knowledge repositories are created and maintained by different communities. They have enabled major advances in terms of data integration, pathway discovery, and machine reading. As powerful as they are, they would be even more useful if they could be linked to representations of everyday knowledge, to better understand the context of texts, and to expressive representations of biomedical theories and the evidential links between theories and experiments.
Many government agencies have been investing in well-scoped areas to create specialized knowledge networks, but fusing these small islands requires enormous effort at the touching points where key integration and innovation projects reside. Open knowledge repositories would provide a semantic infrastructure that would build upon and significantly enlarge these existing limited capabilities. Key steps in achieving this vision include expanding representations for expressive knowledge and developing advanced reasoning and inference methods to operate over these representations.
Heterogeneous Knowledge
Knowledge bases express beliefs about the world, in machine-understandable form, to support multiple uses. For example, billion-fact knowledge bases are used to recognize entities in web search engines, and thereby improve precision. (Every time you see a sidebar box in a web search, or a question answered directly, you are seeing their knowledge bases in use.) Knowledge bases are also used in reasoning systems, natural language understanding, and interactive task learning systems. Some are built by hand and some are built by machine reading. Most open and proprietary resources currently focus on facts about specific entities, such as the population of a town or which actors starred in what movies. These facts are stated via relations that link two or more entities (e.g., a country is linked to the city that is its capital). These kinds of facts are the easiest type of knowledge to extract from texts and databases, and they constitute a major part of human knowledge. But people know much more than just facts, and AI systems need to as well. This section outlines several key types of more expressive knowledge that need to be explored to create integrated intelligent systems. In all cases there has been some progress, but much more research is needed.
An important kind of knowledge are cultural norms, which include etiquette, conventions, protocols, and moral norms, such as prohibitions against murder. Including information about cultural norms is crucial for AI systems to understand not only how to use things but also what is required, permitted, and forbidden. In people, norms are often tacit, which can lead to serious cross-cultural misunderstandings. In AI systems, such knowledge needs to be contextualized, so that the norms of many cultures can be expressed, which will support perspective-taking by AI systems. Another important type of knowledge is information about what things look like, sound like, and even taste and smell like, which helps ground AI systems in the everyday world that we all experience. Understanding the significance of particular types of clothing worn by people, for example, is important in order for machines to understand the world around them.
Decades of research in cognitive science indicate that causality is a key component of human conceptual structure from infancy, and that it is multifaceted. In medicine and biology, causality is tightly tied to statistics, with careful argumentation from trials needed to disentangle causality from correlation. To assist or automate such reasoning will require representing these ways of establishing causality. In commonsense reasoning about the physical and social world, qualitative representations have been developed to provide frameworks for causal knowledge, but these have not yet been applied at scale. In engineering problem solving research, qualitative models have also been used to capture aspects of professional reasoning in several domains, but, again have not yet been explored at scale. Causality in social cognition requires models of human psychology and theory-of-mind reasoning that can represent the beliefs, desires, and intentions of other agents.
Another arena where causality is crucial is in understanding processes, plans, and actions. While AI planning techniques are already richly developed, the focus has mostly been on actions taken by an agent or set of agents, rather than agents continually interacting in a rich world where knowledge is required to operate. AI systems that understand how a business works well enough to participate in evaluating courses of action in response to a crisis, for example, would require deeper understanding of processes, plans, and actions. There is already research on gathering and sharing how-to knowledge for robots, which may suggest a cooperative model for knowledge capture that could be used more broadly. Reasoning about how things work occurs in everyday life, as in trying to figure out what is wrong with a car. Being able to express alternative hypotheses about what is wrong, compute the implications of these hypotheses, and make observations to settle the questions raised are essential aspects of troubleshooting.
Stretch goals: By 2040, our scientific understanding of causal models will be broad and deep enough to provide formalisms that can be used across diverse application areas, including education, medicine, eldercare, and design. A repository of causal models that covers both everyday phenomena and expert performance will enable AI systems to act as collaborators in these domains. Milestones along this path include—
5 years: Causal models at the level of elementary school science knowledge will be used for linking evidence and open questions in science and design, and for supporting advanced decision making.
10 years: Causal models at the level of high school science knowledge in several scientific domains (e.g., biomedicine) will enable AI systems to read and analyze scientific papers, and will support decision making in eldercare support and design.
15 years: Causal models for multiple professional domains will support AI systems working as collaborators with human users.
Diversified Use and Reasoning at Scale
Reasoning puts knowledge to work in answering questions, solving problems, and troubleshooting. Broadly speaking, reasoning combines existing statements to create new ones. Multiple types of human reasoning have been captured to varying degrees in machine reasoning systems. Rarely is one form of reasoning sufficient for a complex task. For example, working out the geopolitical implications of a policy change can involve reasoning about economics, geography, and human motivations. In addition to progress on specific types of reasoning, we must better understand how to make them interoperate flexibly, so that their strengths and weaknesses complement each other to provide better performance and more accurate conclusions in wider ranges of circumstances.
Everyday cultural knowledge is estimated to require in the range of 109 facts, which is orders of magnitude larger than the knowledge bases that most reasoning systems have been used with to date. One of the most difficult challenges will be scaling up reasoning services to operate at that level. Part of the solution is likely to include reformulating open tasks into a set of more tightly constrained problems. For example, the industrial use of satisfiability solvers and model checkers in design indicates that, even when reasoning methods have exponential complexity, they can still be successfully used on practical problems. But the reasoning of model formulation today mostly resides in the minds of the people using those tools. Another tool for scale that people use is reasoning by analogy. By retrieving relevant experiences, long inference chains can be avoided. Statistical reasoning can also help, with metaknowledge providing estimates of utility of approaches and accuracy of conclusions, to enable systems to focus on the most productive reasoning paths. Today’s methods of integrating statistical and relational knowledge vary in degree of formal integration; to date, none of the more formal methods scale to real-world problems.
While people do reason deductively sometimes, evidence from cognitive science indicates that non-deductive reasoning plays a major role in human cognition. One important form of non-deductive reasoning is abduction, which is reasoning to the best explanation. For example, if the windshield of a car is wet, it could be because it is raining, or because its path took it into an area where sprinklers were in use. Abductive reasoning is heavily used in scientific reasoning and troubleshooting, but has been difficult to scale up. Another form of non-deductive reasoning is induction, which makes general conjectures from specific instances. For example, if you see that several cars have speedometers, then you might conjecture that all cars have speedometers. There has been research on a variety of inductive algorithms in machine learning. When the data consists of entities represented by a well defined set of individual attributes (which can be viewed as a simple special case of ground facts), these systems can operate successfully at industrial scale. Induction methods that work with more relational representations (i.e., statements connecting multiple entities) have been explored in limited contexts in inductive logic programming and other areas. Progress in this area is especially important, since relational representations are required for many forms of human knowledge (e.g., plans, explanations, and theories).
Analogy is a third form of non-deductive reasoning that handles relational representations. Analogy, including the particular set of techniques known as case-based reasoning, involves reasoning from one example to another. For example, if trucks are like cars and cars have engines, then one might conjecture that trucks have engines, too. Analogical inferences can be used for explanation as well as prediction. Moreover, analogical generalization, where multiple examples are combined by analogy to yield probabilistic structural representations, provides a form of induction. For example, if someone observes many examples of vehicles on the road, they start to build up models of different kinds of vehicles (e.g., cars and trucks). Evidence from cognitive science suggests that people rely heavily on analogy in their everyday reasoning, and heavy reliance on analogy may help explain why human learning is so much more data-efficient than today’s machine learning techniques. While there are models of matching, retrieval, and generalization that have been used to explain aspects of human reasoning and learning, and applied in several medium-scale domains, today’s accounts of analogical reasoning and learning have yet to be tested at scale—something that open knowledge repositories will enable.
Stretch goals: By 2040, we will have a deep scientific understanding of the integration issues involved in different forms of reasoning, and AI systems will be able to combine them to achieve desired accuracy/performance tradeoffs. A suite of standard, open-source methods for integrating reasoning, using off-the-shelf deductive and non-deductive systems, will be used to rapidly build new AI systems. Milestones along this path include—
5 years: Robust multimodal reasoning will be possible across million-, and billion-fact knowledge bases to carry out decision-support and design tasks.
10 years: Inductive and analogical techniques will incrementally build up and maintain models of tens of thousands of concepts, from hundreds of examples per concept.
15 years: AI systems will be able to understand long complex sequences of events with many actors (e.g., a movie) through analogy and abduction.
Knowledge Capture and Dissemination
Human intelligence is fueled by knowledge accumulated over time by individuals and societies. Similarly, AI systems need to be fueled by knowledge repositories that store the information required to learn or reason about the world. While some of the human knowledge has already been documented and stored in distributed raw or semi-structured documents, there is also knowledge where the people themselves are the main medium of preservation. For example, a major goal for AI has been the accumulation of commonsense knowledge repositories, but to date commonsense knowledge is still largely a human construct. Human knowledge continues to grow due to scientific advances, events (e.g., an election), creativity (e.g., new songs or buildings), discoveries (e.g., a new archeological site), etc.
Several online knowledge sources exist that are quite comprehensive, though never complete. They are semi-structured in nature, or follow certain templates. Examples of such repositories include crowdsourced encyclopedias such as Wikipedia, DBPedia, or Wiktionary, or vertical websites that include specialized collections such as “How To” step-by-step instructions, recipe websites, course offerings for colleges, travel websites, and others. These sources are not directly machine-understandable, that is, in a format that AI systems can directly use. Much of the research work done to extract knowledge and represent it in machine-understandable formats, though, has been focused around using patterns to extract specific types of information.
Important information is often structured in tables or item lists, though the entries and items may still need some processing. For example, a table with basketball players for a team may include their names, but there may be two players named “Maria Smith.” A variety of approaches have been developed for information extraction that take such semi-structured sources and generate knowledge bases that are machine-understandable. An important future challenge is knowledge curation and maintenance over time. Several of these sources are highly dynamic (e.g., Wikipedia), with periodic new contributions and changes. New approaches that can track such temporal changes and update the knowledge repositories to be consistent with the changes and capture the nuances the validity of each piece of knowledge (e.g., if a football player leaves the Miami Dolphins in 2019 then they were a Dolphins player in 2018 but not in 2020) are needed.
The vast majority of human knowledge is stored in raw documents, in the form of text, images, videos, sounds, and other kinds of formats that have no particular structure. Although effective approaches have been developed to extract target categories of entities (e.g., colors or animals), more research is needed to extract other kinds of entities and relations, and to extract knowledge from other media, including images, videos, sounds, and tables.
Recent years have also witnessed a significant increase in the use of crowdsourcing to create knowledge repositories for AI systems. Crowdsourcing and information extraction are often used jointly, where crowdsourcing can fuel automated text extraction, and automated text extraction output can be corrected through crowdsourcing. The quality of the results obtained through crowdsourcing can be improved using a variety of techniques (e.g., by avoiding spam contributions), and knowledge collection pipelines can be used divide the task into smaller working subtasks and subsequently effectively combine their outputs.
Important areas of future research include evaluating the quality and trustworthiness of knowledge repositories, improving them, resolving inconsistencies in the knowledge sources or in the knowledge base, updating the knowledge over time, and reasoning over such knowledge to answer questions.
Stretch goals: By 2040, we will have the ability to create knowledge repositories on target domains or topics as needed by AI systems. Given a target domain, we will be able to identify the sources needed to create such knowledge bases, and extract entities and the relations between them. We will also have algorithms that will validate and maintain these knowledge repositories. We will also have the ability to extract significant amounts of commonsense knowledge from text, images, videos, and other unstructured formats. Milestones along this path include—
5 years: Automatic creation of knowledge bases from raw data, covering different media (text, images, tables, etc.). A suite of methods for creating effective crowdsourcing pipelines for knowledge base construction, sometimes in close interaction with algorithms.
10 years: Knowledge repositories that continuously grow through extraction from sources. Large commonsense knowledge repositories. Algorithms that can effectively validate and maintain very large knowledge repositories.
15 years: AI systems that can understand their own needs in terms of knowledge, and can identify the required sources and construct the knowledge bases they need. Methods for effective reasoning over knowledge bases.
Knowledge Integration and Refinement
The days of a single human, or a team of humans, having a complete understanding of an entire large-scale knowledge base are already over. Given that today’s large-scale knowledge bases are already at 109 facts to cover a reasonable subset of common cultural knowledge, it would not be surprising for extending coverage to include multiple domains of professional knowledge and multimodal knowledge to lead to a total size of 1010 facts. Moreover, most of the new knowledge will be gleaned by AI systems learning, either by reading, watching, or interacting with people. This makes it inevitable that inconsistencies and issues can creep in. Thus, we need to develop reasoning processes that help with the integration of large amounts of knowledge.
Knowledge refinement processes will need to integrate feedback from systems that use knowledge from the repositories, to gather the data needed to diagnose and repair knowledge structures. One difficulty is that there will be a variety of such systems, with different levels of engineering quality, whose properties need to be considered during diagnosis. Moreover, the existence of bad actors, which may try to poison the system by providing misleading reports, also needs to be taken into account.
Stretch goals: By 2040, a scientific understanding of reasoning processes will support knowledge base curation, maintaining accuracy even in the face of low-quality and adversarial inputs. A suite of open-source reasoning processes will enable open knowledge repositories to grow past 109 facts. Milestones along this path include—
5 years: Tools and techniques for knowledge integration will work with expert human curators to build out everyday and professional knowledge in multiple practical domains.
10 years: Tools and techniques for knowledge integration will be semi-autonomous, relying on human experts only when needed or requested, and will be capable of handling adversarial inputs.
15 years: Tools and techniques for knowledge integration will be sufficiently reliable that humans will only be called in a few times per year, by the knowledge service itself or by its users.
3.1.6 Understanding Human Intelligence
Human intelligence is studied not just by AI researchers but also in many other disciplines, such as psychology, linguistics, neuroscience, philosophy, and anthropology. As in AI, these disciplines have mostly focused on particular phenomena, rather than developing integrated accounts of cognition. Cognitive science was formed with the idea that the computational ideas that AI was using to study intelligence might become a language that could also be used to understand minds. The research involved in integrated intelligence that is discussed above will provides a unique opportunity to deepen that connection, using the study of artificial intelligence to inform our theories of natural intelligence.
AI Inspired by Human Intelligence
Work on cognitively and neutrally inspired AI has to date produced two of the most dramatic successes in the history of artificial intelligence: rule-based expert systems that capture high-level articulable patterns and relationships, and neural-network-based deep learning systems that capture low-level non-articulable patterns and relationships. As we turn our sights toward the future, a critical challenge is to understand how humans effectively combine both high-level articulable and low-level non-articulable capabilities to leverage the strengths of each while offsetting the weaknesses of the other, yielding flexible, effective, transparent, and efficient integrated reasoning.
A second challenge is to expand the range of machine learning capabilities to span the myriad of ways in which humans learn from heterogeneous inputs and experiences in order to improve the scope, accuracy, and speed of both individual learning abilities and of the overall system.
A third key research challenge is learning from (and ultimately replicating) how humans reason beyond their local conceptual contexts, which allows them to exhibit critical global thought processes that can be characterized as out-of-the-box creativity, analogy, and the distal transfer of learned knowledge.
A fourth key topic concerns social cognition (as mentioned above), which will enable AI systems to model other intelligent systems—both natural and artificial—so that they can produce individual and group behaviors that are more appropriate and effective than thinking in isolation. These modeling capabilities will need to incorporate adversarial reasoning: As AI systems become ever more broadly deployed and have ever greater impact on human lives, people will inevitably attempt to manipulate them for their benefit, for example by submitting unrepresentative data to machine learning algorithms. AI systems will thus need to be able to reason strategically in order to be robust in the face of such manipulation.
Finally, metacognition is perhaps the least well understood human ability. Part of that capacity is likely due to the flexibility and heterogeneity of knowledge representation in various brain modules, especially the capacity to combine symbolic and statistical information and access it in flexible, introspective ways. Areas most directly involved in metareasoning and reflection include structures of the anterior prefrontal cortex, whose recent development is most characteristic of human evolution, responsible for building, managing, maintaining, and reasoning about goals.
Solutions to no one of these challenges will be sufficient to provide the most comprehensive forms of integration found in the study of human intelligence: Creating this level of artificial intelligence will require cognitive architectures that embody hypotheses concerning more comprehensive combinations of necessary and/or sufficient capabilities. Lessons from such architectures can potentially inform solutions to the individual challenges, while also highlighting possible paths for integration across these areas.
Stretch goals: By 2040, AI systems will exhibit effective human-like integrated adaptive low-level and high-level thinking in complex social environments. Milestones along this path include—
Milestones
5 years: AI systems employ cognitive models that incorporate the full strengths of high-level articulable reasoners, planners, and problem solvers with low-level non-articulable inference networks and learners.
10 years: AI systems use cognitive models that combine a broad spectrum of high-level and low-level learning mechanisms.
15 years: AI systems based on cognitive models that incorporate strong models of the cognitive and social aspects of people and other agents, individually and in groups.
AI to Understand Human Intelligence
A wide range of capabilities in human intelligence have been studied in AI, including symbolic and probabilistic processing, reinforcement learning, and artificial neural networks. Much of this work was originally inspired by models of brain structures and processing. AI has, in turn, led to important insights about the nature of human intelligence. This virtuous cycle of reciprocal benefits that highlights the ideal of what is possible with bidirectional influences.
An area in which this synergy has been particularly notable is the study of learning algorithms. Reinforcement learning has become one of the most important machine learning algorithms in AI. In the brain, it has long been associated with subcortical structures such as the basal ganglia that control the procedural aspect of our behavior, from internal operations that route and request information across brain areas to external actions, including active perception and motor actions. Detailed work has mapped specific aspects of reinforcement learning algorithms onto brain mechanisms such as neurotransmitters. Hebbian learning, initially identified as a principle for local learning at the synaptic level, has led to AI algorithms such as backpropagation that have been central to deep learning. New principles of Hebbian learning, such as spike-timing-dependent plasticity, hold the promise of further advances in learning algorithms that are more robust and self-regulating. More broadly, the study of how those processes operate in concert within the complex structure of the human brain can shed light on how to integrate a variety of learning algorithms in complex AI systems.
Stretch goals: By 2040, broad-coverage integrated models of mind and brain will be applied in concert to enable intelligent reasoning spanning time scales from one millisecond to one month. Milestones along this path include—
Milestones
5 years: AI systems could be designed to study psychological models of complex intelligent phenomena that are based on combinations of symbolic processing and artificial neural networks.
10 years: Integrated architectures are the standard vehicle for modeling the results of complex psychological experiments.
15 years: Progress in AI on neural networks and integrated architectures yields major advances in neural/brain modeling.
Towards Unifying Theories of Natural and Artificial Intelligence
Ultimately, a comprehensive theory of integrated intelligence is desirable that spans all possible forms of intelligence, whether natural or artificial. Such a grand challenge will likely take much more than 20 years to complete, but a start can be made within this time frame by focusing on more modest goals. Recently, a new community has begun to coalesce around the goal of designing human-like cognitive architectures that model both human and artificial intelligence. Among other things, such a common architectural framework could provide a useful intermediary in evaluating and comparing cognitive architectures and create a pathway for unifying models of natural and artificial intelligence.
An essential aspect of those architectures that has increasingly guided their development is the ability to validate them using neural imaging data. Neural imaging data, assembled in large databases, such as the Human Connectome Project covering substantial subject populations performing a diverse range of tasks and using a number of imaging techniques, provides constraints regarding both the structural and functional organization of brain modules as well as the details of knowledge representation within those modules. This new source of data expands on the wealth of existing behavioral data accumulated over more than a century of research, provides converging evidence for and against proposed architectures, and holds the promise to considerably speed up convergence to a consensus theory of natural and artificial intelligence.
Stretch goals: By 2040, a common cognitive architectural model will yield deep understanding across at least one full arc of cognition, from perception to behavior, for a complex task in a real environment. Milestones along this path include—
Milestones
5 years: The full space of existing cognitive architectures (i.e., integrated models of human-like intelligence) is mapped onto a single common model of cognition.
10 years: Strong connections are demonstrated between AI architectures and cognitive models that can be mapped at the level of major brain regions, their functional connectivity and mechanisms, and their communication patterns.
15 years: Shared implemented models of cognition are in wide use by both the AI and computational cognitive science communities.
Full Report
Table of Contents:
Title Page, Executive Summary, and Table of Contents (7 pages)
1. Introduction (5 pages)
2. Major Societal Drivers for Future Artificial Intelligence Research (4 pages)
3. Overview of Core Technical Areas of AI Research Roadmap: Workshop Reports
- Workshop I: A Research Roadmap for Integrated Intelligence (19 pages)
- Workshop II: A Research Roadmap for Meaningful Interaction (27 pages)
- Workshop III: A Research Roadmap for Self-Aware Learning (26 pages)
4. Major Findings (4 pages)
5. Recommendations (22 pages)
6. Conclusions (1 page)
7. Appendices (participants and contributors) (2 pages)